· Charlotte Will · webscraping · 5 min read
How to Scrape Data from Multiple Pages with Python
Discover how to efficiently scrape data from multiple pages using Python, automating data extraction and enhancing your web scraping skills. Learn about handling pagination, optimizing requests, and storing data in structured formats. This comprehensive guide covers both basic and advanced techniques for successful web scraping projects.
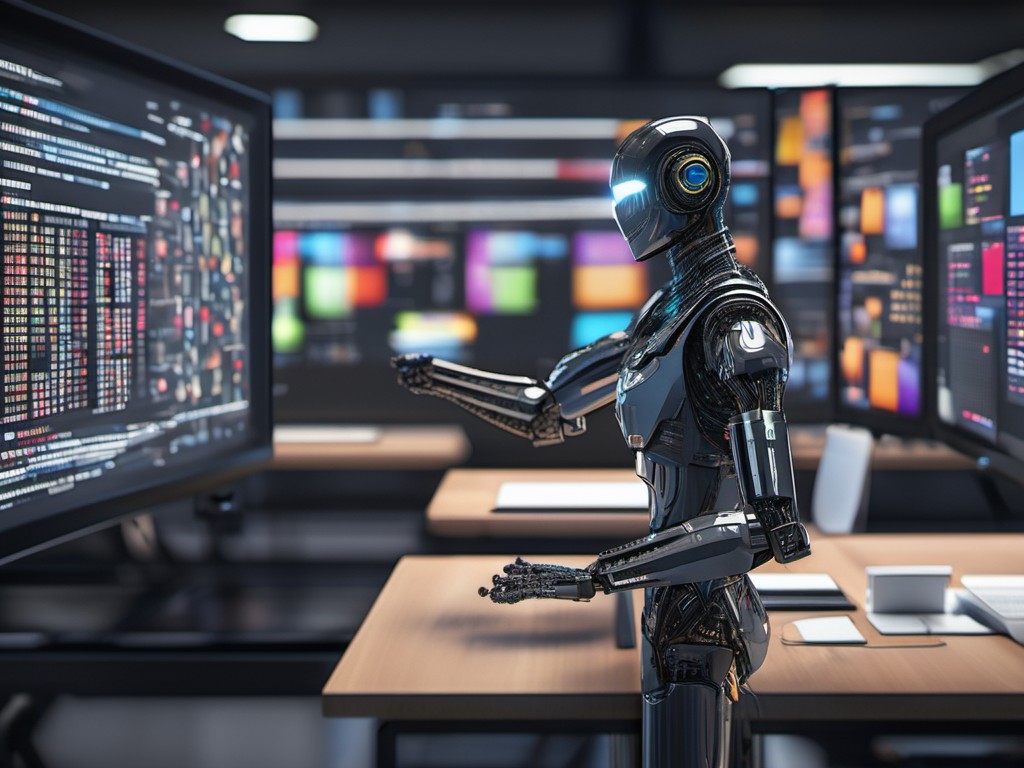
Web scraping is an essential skill in today’s data-driven world, allowing you to extract valuable information from websites efficiently. When dealing with multiple pages, the process can become more complex but also highly rewarding. This comprehensive guide will walk you through how to scrape data from multiple pages using Python, ensuring you can automate data collection effectively.
Why Scrape Data from Multiple Pages?
Imagine trying to gather product information from an e-commerce site that lists thousands of items across hundreds of pages. Manually copying and pasting this data would be time-consuming and error-prone. Python web scraping allows you to automate this process, saving you countless hours and ensuring accurate data extraction.
Prerequisites
Before diving into the code, make sure you have the following tools installed:
- Python (preferably version 3.x)
- Pip (Python package installer)
- Required libraries:
requests
,BeautifulSoup
, andpandas
You can install these libraries using pip:
pip install requests beautifulsoup4 pandas
Basic Web Scraping with Python
Let’s start by scraping data from a single page to understand the basics. We’ll use requests
to fetch the webpage and BeautifulSoup
for parsing HTML content.
Fetching the Webpage
import requests
url = 'https://example.com/page1'
response = requests.get(url)
webpage_content = response.text
Parsing HTML Content
from bs4 import BeautifulSoup
soup = BeautifulSoup(webpage_content, 'html.parser')
print(soup.prettify())
Scraping Data from Multiple Pages
Now that you’re comfortable with basic web scraping, let’s move on to handling multiple pages. The approach involves:
- Fetching the first page to understand its structure.
- Identifying pagination links or patterns.
- Iterating through each page and extracting data.
- Storing the extracted data in a structured format like a CSV file using
pandas
.
Step-by-Step Guide
1. Fetching the First Page
url = 'https://example.com/page1'
response = requests.get(url)
webpage_content = response.text
soup = BeautifulSoup(webpage_content, 'html.parser')
2. Identifying Pagination Links or Patterns
Inspect the webpage to find pagination links. These are usually in the form of <a>
tags with specific classes or IDs.
3. Iterating Through Each Page and Extracting Data
Let’s assume we’re scraping product data, including title and price. Here’s a function to extract this information from each page:
def extract_product_data(page_content):
soup = BeautifulSoup(page_content, 'html.parser')
products = []
for item in soup.select('.product-item'): # Adjust the selector based on the site's HTML structure
title = item.select_one('.title').text
price = item.select_one('.price').text
products.append({'title': title, 'price': price})
return products
4. Automating the Process
We’ll create a loop to iterate through each page and extract data:
base_url = 'https://example.com/page'
pages = range(1, 11) # Adjust the range based on the number of pages
all_products = []
for page in pages:
url = f"{base_url}{page}"
response = requests.get(url)
webpage_content = response.text
products = extract_product_data(webpage_content)
all_products.extend(products)
5. Storing Data in CSV Format
Finally, we’ll store the extracted data in a CSV file using pandas
.
import pandas as pd
df = pd.DataFrame(all_products)
df.to_csv('product_data.csv', index=False)
Scraping Data Efficiently Using Python
To enhance efficiency, consider these tips:
- Use Headless Browsers: For dynamic content, tools like Selenium or Playwright can render JavaScript and fetch data more accurately.
- Implement Error Handling: Add try-except blocks to handle potential issues like network errors or changes in webpage structure.
- Optimize Requests: Use session objects with
requests
to maintain persistent connections, reducing latency. - Respect Robots.txt and Terms of Service: Ensure you comply with the website’s policies to avoid legal issues.
For more insights on efficient data extraction, refer to our detailed guide: How to Scrape Data Efficiently Using Python.
Automate Data Collection with Python Webscraping
Automating data collection can save you time and effort. By setting up a scheduled task or using cloud services, you can ensure continuous data extraction without manual intervention.
Learn more about automating data collection: Automating Data Collection with Python Webscraping.
The Importance of Python Webscraping in Data Analysis
Data scraped from multiple pages can be invaluable for various data analysis tasks. From market research to competitive analysis, having structured data at your fingertips can provide actionable insights.
For deeper understanding, explore: The Importance of Python Webscraping in Data Analysis.
Conclusion
Scraping data from multiple pages with Python is a powerful technique that can save you time and effort. By understanding the basics, identifying pagination patterns, and automating the process, you can efficiently extract valuable information. Whether you’re gathering product details or conducting market research, the skills you’ve learned in this guide will serve you well.
FAQs
What are some common issues faced when scraping data from multiple pages?
Common issues include changes in webpage structure, rate limiting by servers, and handling dynamic content loaded via JavaScript. Implementing error handling and using headless browsers can help mitigate these problems.
How do I handle rate limiting while scraping multiple pages?
Rate limiting refers to the server’s restriction on the number of requests you can make within a certain timeframe. To handle this, you can introduce delays between requests or use rotating proxies to distribute your requests across different IP addresses.
Can I scrape data from websites that require login?
Yes, it is possible to scrape data from sites requiring login by simulating the login process using tools like Selenium or Playwright. However, ensure you comply with the site’s terms of service before proceeding.
What are headless browsers and why are they useful in web scraping?
Headless browsers are browser instances that operate without a graphical user interface. They are useful for web scraping because they can render JavaScript-heavy content, providing a more accurate representation of the page’s data compared to simple HTTP requests.
How do I ensure my web scraper is not violating any laws or terms of service?
To ensure compliance with laws and terms of service:
- Always review the site’s
robots.txt
file to understand which pages are off-limits for scraping. - Check the website’s terms of service for any restrictions on automated access.
- Avoid scraping sensitive or personal data without explicit permission.